Artificial Intelligence-Based Approach for Hybrid Assembly Line Preliminary Design in the Automotive Industry
CIFRE Ph.D. Thesis - FORVIA/Faurecia & University of Franche-Comté, FEMTO-ST Institute, CNRS (2024)
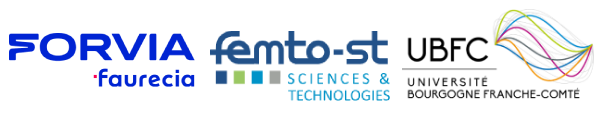
To be defended between December 2024 - February 2025.
Jury Members:
TBD | TBD | TBD | President |
TBD | TBD | TBD | Rapporteur |
TBD | TBD | TBD | Rapporteur |
TBD | TBD | TBD | Examiner |
Julien BOURGEOIS | Professor | University of Franche-Comté | Thesis Director |
Raphael DE MATOS | Digital Manager | FORVIA Clean Mobility | Company Manager |
In the dynamic landscape of the automotive industry, the imperative for innovative and efficient assembly line design is crucial. This doctoral thesis introduces a novel framework based on Artificial Intelligence for the automation and acceleration of the preliminary design of hybrid assembly lines in the automotive industry. The overarching goal is to revolutionize the conventional design process, hastening development, improving operational efficiency, and ultimately reducing time to market.
The research is structured into three integral parts, each addressing pivotal aspects of assembly line design. The first segment focuses on the meticulous optimization of assembly scenarios and task/machine allocation. Utilizing metaheuristic algorithms, this section dissects the intricate details of assembly scenarios, ensuring an optimal arrangement of tasks and machines. By employing advanced optimization techniques, the framework aims to streamline the assembly process, minimizing bottlenecks, and enhancing overall productivity.
The second part of the framework emphasizes resource optimization, specifically targeting the reduction of machines and operators. Rooted in reinforcement learning techniques, this phase aims to construct a globally optimized sequence that adheres to company rules and accommodates stakeholder preferences. Through a careful balance of efficiency and resource minimization, the framework aims to create assembly lines that are not only cost-effective but also tailored to meet the unique requirements of the automotive manufacturing environment.
The third and final part of the thesis addresses the dynamic nature of assembly lines, tackling challenges such as breakdowns, hazards, buffer limitations, idle times, learning models and operator fatigue. This section integrates predictive modeling and stochastic approaches to anticipate and mitigate disruptions, fostering adaptability and resilience in the face of real-world manufacturing uncertainties. The inclusion of client constraints ensures that the designed assembly lines align with the specific needs and expectations of end-users.
The holistic framework proposed in this thesis signifies a paradigm shift in assembly line design. By integrating state-of-the-art AI techniques, it not only expedites the design process but significantly enhances the efficiency and flexibility of automotive manufacturing. The outcomes of this research hold the promise of a transformative impact on the industry, providing a sophisticated toolset to designers and manufacturers striving to stay competitive in the automotive market. This AI-driven approach serves as a testament to the potential of technology to reshape traditional paradigms, enabling the creation of assembly lines that are not only optimized but also adaptive to the dynamic challenges of contemporary manufacturing.